Practical Cases
Environmental Quality
Prediction
Technology
Using Deep Learning
Environmental Quality Prediction
Technology Using
Deep Learning
Aiming to realize environmental smart services
through AI-based IoT data collaboration
Keywords: AI, Environmental quality prediction, Cross-data analysis
Overview
We are conducting research and development on a deep learning methods for discovering and predicting spatial and temporal association patterns in heterogeneous sensing data related to the urban environment and applying them to the short-term prediction and behavioral support of the effects of abnormal weather and air pollution on social life (environmental quality), such as traffic and health. By devising a prediction model inconsideration of the spatial and temporal continuity and locality of the association pattern, both high versatility and prediction accuracy are achieved. We are also working on the development of technology to optimize predictions by linking user-collected data.
Possibility of utilizing results
This technology has been implemented in the xData platform on NICT’s Integrated Testbed, and is being applied to support early warning and alert issuance for air pollution, smart services for environment-aware mobility and healthcare, etc. We are also engaged in user-participatory development of environmental quality prediction models and smart services. In addition, we are conducting collaborative research both in Japan and overseas to reuse, share, and transfer environmental quality prediction models.
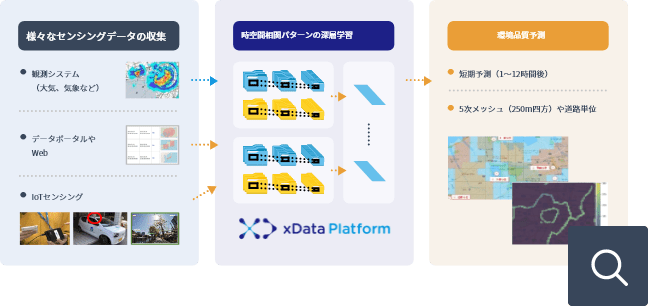
-
Heterogeneous sensing
data collection -
Deep learning for spatial &
temporal association patterns -
Environmental quality
prediction
Application examples
-
Photochemical Oxidant Warning・Early Warning Support for Warning Issuance
-
Traffic Pollution Risk Prediction
-
Prediction of Damage from Smog Transboundary Pollution
Information Assets
References
- Peijiang Zhao, Koji Zettsu: Decoder Transfer Learning
for
Predicting
Personal Exposure to Air
Pollution. BigData 2019: 5620-5629. - Koji Zettsu: Transforming Sensing Data into Smart Data for Smart Sustainable Cities. BDA 2019: 3-19